The demand for skilled AI professionals is increasing rapidly. In fact, according to recent reports, the average salary for AI professionals in the United States is over $100,000 per year, with some specialized roles commanding even higher salaries.
Check Out My NO-1 Proven Method to Earn $100-$500/Day with FREE Traffic
One popular AI method that has been gaining traction in recent years is the use of paid AI models. These models, which are often developed by large tech companies like Google and Microsoft, use advanced algorithms and machine learning techniques to analyze large amounts of data and provide valuable insights to businesses.
- Accuracy: Paid AI models are often more accurate than other AI methods because they have been developed and trained using vast amounts of high-quality data. This means that they can provide more precise predictions and insights, which can be invaluable for businesses looking to make informed decisions.
- Customizability: Many paid AI models can be customized to meet the specific needs of a business. This means that businesses can choose the parameters that the model uses to analyze data, ensuring that the results are relevant and useful.
- Speed: Paid AI models can often process data much faster than other AI methods, which can be crucial for businesses that need to make decisions quickly. This is especially important in industries like finance and healthcare, where timely decisions can have a significant impact on outcomes.
- Support: Paid AI models are often developed and supported by large tech companies, which means that businesses can rely on the expertise of these companies to help them implement and optimize the models. This can be especially helpful for businesses that are new to AI and may not have the necessary expertise in-house.
- Cost-effectiveness: While paid AI models can be expensive, they can also be highly cost-effective in the long run. This is because they can help businesses save time and money by automating processes and providing valuable insights that can inform decision-making and improve outcomes.
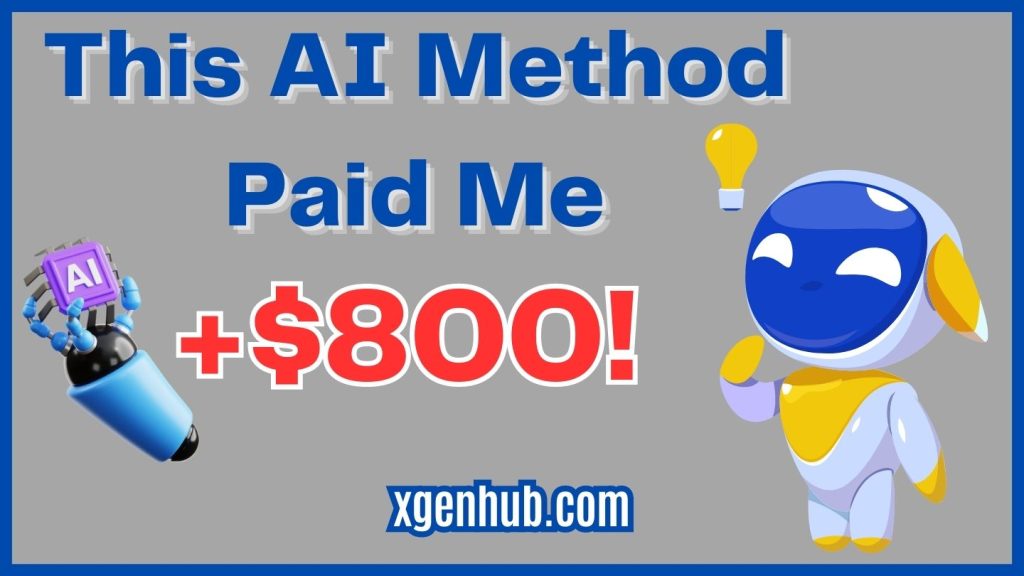
While they may not be the right choice for every business, they are certainly worth considering for those looking to leverage the power of AI to drive growth and success.
Accuracy
Accuracy is a crucial factor in many fields, from science and engineering to finance and healthcare. In the context of data analysis and artificial intelligence (AI), accuracy refers to the degree to which a model or algorithm can correctly predict or classify data points.
Check Out My NO-1 Proven Method to Earn $100-$500/Day with FREE Traffic
- Importance: Accuracy is important because it can impact the effectiveness of decision-making and problem-solving. In fields like healthcare, for example, inaccurate predictions or diagnoses can have serious consequences for patient outcomes. In finance, inaccurate predictions can lead to poor investment decisions and financial losses.
- Metrics: There are several metrics used to evaluate the accuracy of an AI model, including precision, recall, and F1 score. These metrics help quantify the model’s ability to correctly identify true positives, true negatives, false positives, and false negatives.
- Data Quality: The accuracy of an AI model is highly dependent on the quality and quantity of the data used to train it. High-quality data with few errors or biases can help ensure that the model is more accurate in predicting future outcomes.
- Overfitting and Underfitting: Overfitting occurs when a model is too complex and fits the training data too closely, resulting in poor performance on new data. Underfitting, on the other hand, occurs when a model is too simple and does not capture all the relevant patterns in the data. Both overfitting and underfitting can result in lower accuracy.
- Improvement: There are several ways to improve the accuracy of an AI model, including increasing the quality and quantity of the training data, adjusting the model’s parameters, and using more advanced algorithms and techniques like deep learning.
It is important to evaluate and improve the accuracy of AI models through careful attention to data quality, model complexity, and algorithm selection. With accurate AI models, businesses and industries can make more informed decisions, drive innovation, and improve overall performance.
Customizability
Customizability is a critical aspect of many software applications, including artificial intelligence (AI) models. In the context of AI, customizability refers to the ability to tailor the model to meet the specific needs of a particular application or problem.
- Importance: Customizability is essential because it allows businesses to address specific challenges or opportunities in their operations. By tailoring AI models to their unique needs, businesses can gain more valuable insights and make better decisions.
- Flexibility: Customizability allows businesses to modify or add features to an AI model based on evolving business needs or changes in the market. This can help ensure that the model continues to provide relevant insights and stay ahead of the competition.
- Parameters: Customizability in AI models often involves adjusting the model’s parameters. These parameters can include variables such as the size of the model, the types of algorithms used, and the specific features of the data that the model analyzes.
- Data Selection: Customizability can also involve selecting specific data sets to use in training the AI model. By choosing high-quality data sets that are specific to the problem at hand, businesses can increase the accuracy and relevance of the model’s predictions and insights.
- Domain-Specific: Customizability can also involve tailoring AI models to specific domains or industries. For example, an AI model designed for healthcare may require different parameters and datasets than one designed for finance or manufacturing.
Adjusting parameters, selecting relevant data sets, and tailoring models to specific domains or industries, businesses can gain more valuable insights and make better decisions. Customizable AI models can help businesses stay ahead of the competition and drive innovation and growth.
Speed
Speed is a critical factor in many fields, including technology, finance, and healthcare. In the context of data analysis and artificial intelligence (AI), speed refers to the time it takes for an algorithm or model to process data and provide results.
Check Out My NO-1 Proven Method to Earn $100-$500/Day with FREE Traffic
- Importance: Speed is important because it can impact the effectiveness of decision-making and problem-solving. In industries like finance, for example, speed is crucial for making real-time investment decisions. In healthcare, speed can be critical for timely diagnoses and treatments.
- Hardware: The speed of an AI algorithm or model is often dependent on the hardware used to run it. High-performance hardware like GPUs and TPUs can help accelerate computations and speed up the processing of large datasets.
- Optimization: AI models can be optimized to improve their speed by reducing the amount of time it takes to process data. Techniques like batch processing and parallel processing can help speed up computations and reduce overall processing times.
- Data Volume: The speed of an AI model is also dependent on the volume of data it processes. Large datasets can significantly slow down processing times, and techniques like data sampling or feature selection can help reduce the amount of data used in the model.
- Real-Time: For some applications, real-time processing is critical. For example, in industries like finance and transportation, real-time data processing is essential for making quick and accurate decisions. Real-time processing requires high-performance hardware and optimized algorithms to ensure fast and accurate results.
It is important to optimize AI models to improve their processing times, reduce data volume, and ensure real-time processing when necessary. With faster and more accurate AI models, businesses and industries can make more informed decisions, drive innovation, and improve overall performance.
Support
Support is a critical aspect of any technology or software, including artificial intelligence (AI) models. In the context of AI, support refers to the assistance and resources available to users of the model, such as technical support, training, and documentation.
- Importance: Support is essential because it ensures that users can effectively and efficiently use the AI model to achieve their goals. Without proper support, users may struggle to understand or troubleshoot issues, leading to frustration and poor performance.
- Technical Support: Technical support is an essential aspect of AI support. It provides users with assistance when they encounter technical issues with the model or software, such as bugs or errors. Technical support can be provided through various channels, including email, phone, or live chat.
- Training: Training is another essential aspect of AI support. It provides users with the knowledge and skills necessary to effectively use the model to achieve their goals. Training can include resources such as documentation, tutorials, and hands-on workshops.
- Community: A supportive community of users and developers can be invaluable in providing assistance and resources to users of an AI model. Online forums, user groups, and social media can connect users with others who have experience using the model and can provide advice and assistance.
- Updates: Regular updates and improvements to the model can also be considered a form of support. By providing users with the latest features and bug fixes, developers can ensure that users can continue to effectively use the model and stay ahead of the competition.
Technical support, training, community resources, and regular updates can all contribute to effective support for users of an AI model. With proper support, users can achieve greater success with the model, drive innovation, and improve overall performance.
Cost-effectiveness
Cost-effectiveness is a crucial factor to consider when implementing artificial intelligence (AI) models in any industry or organization. Cost-effectiveness refers to the balance between the cost of developing and implementing an AI model and the benefits it provides in terms of efficiency, accuracy, and overall performance.
Check Out My NO-1 Proven Method to Earn $100-$500/Day with FREE Traffic
- Importance: Cost-effectiveness is critical because it ensures that the benefits of implementing an AI model outweigh the costs. AI models can be costly to develop and implement, and it is essential to ensure that the investment is justified by the benefits provided.
- Planning: Effective planning is essential to ensure cost-effectiveness. Organizations must consider factors such as the size and complexity of the data to be analyzed, the hardware required, and the necessary expertise to develop and implement the model.
- Scalability: Scalability is a crucial aspect of cost-effectiveness. An AI model that can be easily scaled to handle large volumes of data can significantly reduce costs and improve efficiency.
- ROI: Return on investment (ROI) is a key metric for assessing cost-effectiveness. It is essential to estimate the potential ROI of implementing an AI model before investing significant resources.
- Open-source: Open-source AI models can be a cost-effective alternative to proprietary models. Many open-source AI models are available for free or at a significantly lower cost than proprietary models and can be customized to meet specific organizational needs.
Effective planning, scalability, ROI analysis, and consideration of open-source alternatives can all contribute to cost-effective AI implementations. With cost-effective AI models, organizations can improve efficiency, accuracy, and overall performance while minimizing costs and maximizing return on investment.
Thank you for taking the time to read my rest of the article, (NEW!!) This AI Method Paid Me +$800! DO It ASAP!